Bibcode
Garcia-Dias, Rafael; Allende Prieto, Carlos; Sánchez Almeida, Jorge; Alonso Palicio, Pedro
Referencia bibliográfica
Astronomy and Astrophysics, Volume 629, id.A34, 14 pp.
Fecha de publicación:
9
2019
Revista
Número de citas
13
Número de citas referidas
13
Descripción
Context. The vast volume of data generated by modern astronomical
surveys offers test beds for the application of machine-learning. In
these exploratory applications, it is important to evaluate potential
existing tools and determine those that are optimal for extracting
scientific knowledge from the available observations. Aims: We
explore the possibility of using unsupervised clustering algorithms to
separate stellar populations with distinct chemical patterns.
Methods: Star clusters are likely the most chemically homogeneous
populations in the Galaxy, and therefore any practical approach to
identifying distinct stellar populations should at least be able to
separate clusters from each other. We have applied eight clustering
algorithms combined with four dimensionality reduction strategies to
automatically distinguish stellar clusters using chemical abundances of
13 elements. Our test-bed sample includes 18 stellar clusters with a
total of 453 stars. Results: We have applied statistical tests
showing that some pairs of clusters (e.g., NGC 2458-NGC 2420) are
indistinguishable from each other when chemical abundances from the
Apache Point Galactic Evolution Experiment (APOGEE) are used. However,
for most clusters we are able to automatically assign membership with
metric scores similar to previous works. The confusion level of the
automatically selected clusters is consistent with statistical tests
that demonstrate the impossibility of perfectly distinguishing all the
clusters from each other. These statistical tests and confusion levels
establish a limit for the prospect of blindly identifying stars born in
the same cluster based solely on chemical abundances. Conclusion. We
find that some of the algorithms we explored are capable of blindly
identify stellar populations with similar ages and chemical
distributions in the APOGEE data. Even though we are not able to fully
separate the clusters from each other, the main confusion arises from
clusters with similar ages. Because some stellar clusters are chemically
indistinguishable, our study supports the notion of extending weak
chemical tagging that involves families of clusters instead of
individual clusters.
The list of stars is only available at the CDS via anonymous ftp to http://cdsarc.u-strasbg.fr
(ftp://130.79.128.5) or via http://cdsarc.u-strasbg.fr/viz-bin/qcat?J/A+A/629/A34
Proyectos relacionados
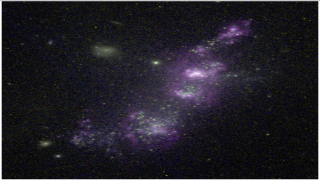
Grupo de Estudios de Formación Estelar GEFE
El proyecto interno GEFE está enmarcado en el proyecto coordinado, ESTALLIDOS, financiado por el plan nacional desde el año 2001. El ultimo proyecto aprobado es ESTALLIDOS 6.0 (AYA2016- 79724-C4-2-P). En el proyecto GEFE trabajamos en base al caso científico del proyecto ESTALLIDOS 6.0. Los estallidos de formación estelar (Starbursts o SB) son
Casiana
Muñoz Tuñón
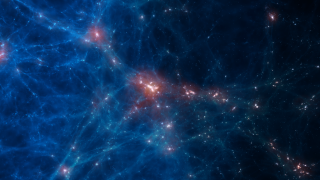
Astrofísica Numérica: Formación y Evolución de Galaxias
Entre las cuestiones fundamentales en Astronomía y Astrofísica están la formación y evolución de galaxias. Las escalas de tiempo y tamaño son tan astronómicas que su observación en galaxias individuales es imposible. Solo con el uso de simulaciones numéricas es posible entender la formación de estructuras cósmicas dentro del actual marco
Claudio
Dalla Vecchia
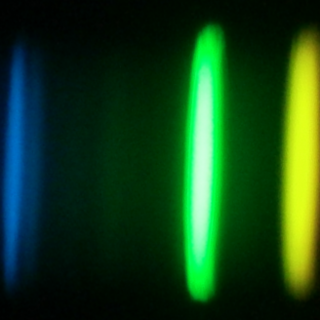
Abundancias Químicas en Estrellas
La espectroscopía de estrellas nos permite determinar las propiedades y composiciones químicas de las mismas. A partir de esta información para estrellas de diferente edad en la Vía Láctea es posible reconstruir la evolución química de la Galaxia, así como el origen de los elementos más pesados que el boro, forjados principalmente en los interiores
Carlos
Allende Prieto