Lecture Notes in Computer Science
Temperature data acquired by infrared sensors provide relevant information to assess different medical pathologies in early stages, when the symptoms of the diseases are not visible yet to the naked eye. Currently, a clinical system that exploits the use of multimodal images (visible, depth and thermal infrared) is being developed for diabetic foot monitoring. The workflow required to analyze these images starts with their acquisition and the automatic feet segmentation. A novel approach is presented for automatic feet segmentation using Deep Learning employing an architecture composed of an encoder and decoder (U-Net architecture) and applying a segmentation of planes in point cloud data, using the depth information of pixels labeled in the neural network prediction. The proposed automatic segmentation is a robust method for this case study, providing results in a short time and achieving better performance than other traditional segmentation methods as well as a basic U-Net segmentation system.
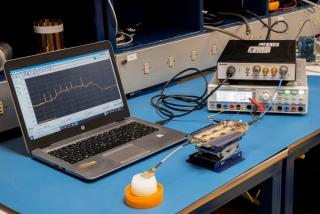