Bibcode
Mong, Y. -L.; Ackley, K.; Killestein, T. L.; Galloway, D. K.; Vassallo, C.; Dyer, M.; Cutter, R.; Brown, M. J. I.; Lyman, J.; Ulaczyk, K.; Steeghs, D.; Dhillon, V.; O'Brien, P.; Ramsay, G.; Noysena, K.; Kotak, R.; Breton, R.; Nuttall, L.; Pallé, E.; Pollacco, D.; Thrane, E.; Awiphan, S.; Burhanudin, U.; Chote, P.; Chrimes, A.; Daw, E.; Duffy, C.; Eyles-Ferris, R.; Gompertz, B. P.; Heikkilä, T.; Irawati, P.; Kennedy, M.; Levan, A.; Littlefair, S.; Makrygianni, L.; Marsh, T.; Mata Sánchez, D.; Mattila, S.; Maund, J. R.; McCormac, J.; Mkrtichian, D.; Mullaney, J.; Rol, E.; Sawangwit, U.; Stanway, E.; Starling, R.; Strøm, P.; Tooke, S.; Wiersema, K.
Referencia bibliográfica
Monthly Notices of the Royal Astronomical Society
Fecha de publicación:
1
2023
Número de citas
3
Número de citas referidas
3
Descripción
Developing an effective automatic classifier to separate genuine sources from artifacts is essential for transient follow-ups in wide-field optical surveys. The identification of transient detections from the subtraction artifacts after the image differencing process is a key step in such classifiers, known as real-bogus classification problem. We apply a self-supervised machine learning model, the deep-embedded self-organizing map (DESOM) to this 'real-bogus' classification problem. DESOM combines an autoencoder and a self-organizing map to perform clustering in order to distinguish between real and bogus detections, based on their dimensionality-reduced representations. We use 32 × 32 normalized detection thumbnails as the input of DESOM. We demonstrate different model training approaches, and find that our best DESOM classifier shows a missed detection rate of $6.6{{\ \rm per\,cent}}$ with a false-positive rate of $1.5{{\ \rm per\,cent}}$. DESOM offers a more nuanced way to fine-tune the decision boundary identifying likely real detections when used in combination with other types of classifiers, e.g. built on neural networks or decision trees. We also discuss other potential usages of DESOM and its limitations.
Proyectos relacionados
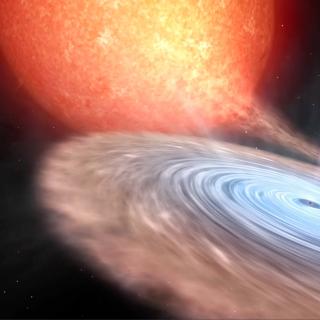
Agujeros negros, estrellas de neutrones, enanas blancas y su entorno local
Los agujeros negros y estrellas de neutrones en binarias de rayos-X son laboratorios únicos para explorar la física de estos objetos compactos. No solo permiten confirmar la existencia de agujeros negros de origen estelar a través de mediciones dinámicas de sus masas, sino que también permiten investigar el comportamiento de la materia y la
Montserrat
Armas Padilla
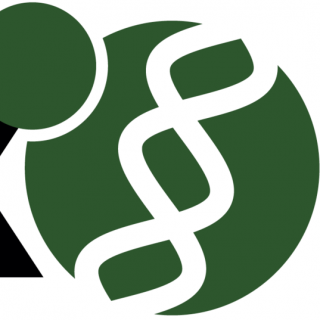
Exoplanetas y Astrobiología
La búsqueda de vida en el Universo se ha visto impulsada por los recientes descubrimientos de planetas alrededor de otras estrellas (los llamados exoplanetas), convirtiéndose en uno de los campos más activos dentro de la Astrofísica moderna. En los últimos años los descubrimientos cada vez más numerosos de nuevos exoplanetas y los últimos avances
Enric
Pallé Bago