Bibcode
Iglesias, F. A.; Asensio Ramos, A.; Sanchez, M.; Feller, A.
Bibliographical reference
Boletin de la Asociacion Argentina de Astronomia La Plata Argentina
Advertised on:
8
2024
Citations
0
Refereed citations
0
Description
Current polarimetric calibration techniques derive the instrument modulation matrix by numerically fitting an instrumental model to measurements of a set of calibration Stokes vectors. These techniques are typically limited to an error on the retrieved normalized Stokes Q, U and V parameters, in the $1\times10^{-2}$ to $1\times10^{-3}$ range. This error commonly increases when the instrument response varies considerably across its field of view and/or when instrumental effects are present, which are not included in the assumed calibration model, such as camera non-linearity. We propose a new technique to calibrate Stokes polarimeters based on a model composed of fully-connected, multi- layer neural networks (NN's). This model is trained to learn the instrument modulation matrix given a set of input parameters, using the same calibration data that is acquired for the current techniques. The main advantage of our NN-based approach is its flexibility to incorporate instrumental effects for which no accurate model is available and, possibly through the fusion of data from other types of relevant calibrations, obtain a more accurate instrument response. We present a preliminary result of our model performance using synthetic data, where the ground truth is known.
Related projects
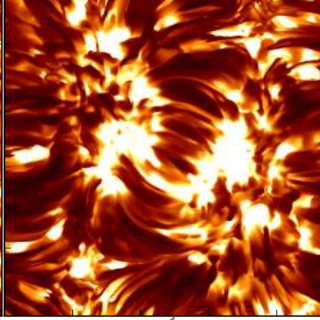
Magnetism, Polarization and Radiative Transfer in Astrophysics
Magnetic fields pervade all astrophysical plasmas and govern most of the variability in the Universe at intermediate time scales. They are present in stars across the whole Hertzsprung-Russell diagram, in galaxies, and even perhaps in the intergalactic medium. Polarized light provides the most reliable source of information at our disposal for the
Tanausú del
Pino Alemán
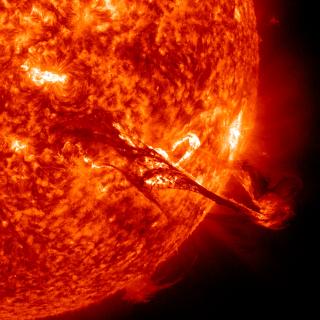
Solar and Stellar Magnetism
Magnetic fields are at the base of star formation and stellar structure and evolution. When stars are born, magnetic fields brake the rotation during the collapse of the mollecular cloud. In the end of the life of a star, magnetic fields can play a key role in the form of the strong winds that lead to the last stages of stellar evolution. During
Tobías
Felipe García